Task
I am interested in working on the math OCR (optical character recognition) problem. According to the Infty project, there are multiple levels of solutions to this task:
- "Level 1: bitmap images of printed materials (e.g. GIF, TIFF, PNG). This is the input to be processed.
- Level 2: searchable digitized document (e.g. PS, PDF),
- Level 3: logically structured document with links (e.g. HTML, MathML,LATEX),
- Level 4: partially executable document (e.g. Mathematica, Maple),
- Level 5: formally presented document. (e.g. Mizar, OMDoc)"
Dataset Processing
- I have been completely using the Infty dataset for analysis. The set consists of 30 mathematical documents on the order of 10 pages each.
- I have trimmed down the csv files to only the information I need, and removed odd labellings.
- I have written a Matlab script to extract all of the labelled characters and their corresponding labellings (an id which corresponds to latex and mathml representations).
Preprocessing
- This took the bulk of my effort, which is what I anticipated.
- My original goal in preprocessing was to extract parametric polynomial skeletal curves for parts of the characters. I found this to be a difficult task, and found that a sequence of constant-length line segments would give better results faster.
- I extract chains of line segments in the following manner:
- Choose randomly two black points, move one of the points closer to the other until the length is as given (ie scale their difference vector).
- Adjust this original segment to be as close to the center of "blackness" as possible.
- Add a point to the chain, and adjust this segment as in 2.
- Repeat on both sides of the original segment.
- The results produced the chains I wanted, as shown below:
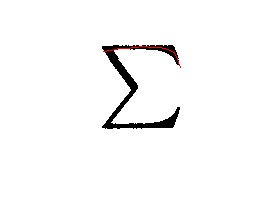
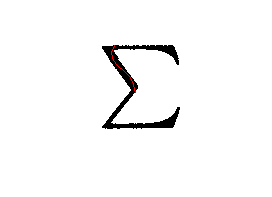
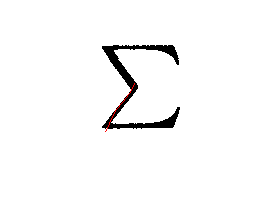
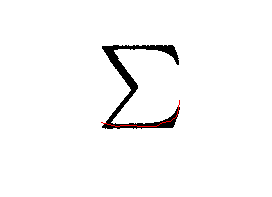
Plsa
- I have begun implementing plsa in Matlab, and I have found a matlab implementation online. I may supplement my implementation with parts of the implementation I found, or possibly parts of a faster C implementation.
- My plan for linking my preprocessing to plsa is very simple as of now:
- Extract random chains (let's say 10 chains) for each character.
- For each chain:
- For each segment of each chain I have been able to find the standard deviation of near black points from the segment. This gives the "width" of the segment.
- Find the "widths" of the segments in the chain.
- Find the angle between each segment and the previous segment
- Reduce each of the above two vectors into vectors of some prechosen length (let's say 10) by composing and averaging respectively. These combine to form the descriptor for the chain.
- Run kmeans on the descriptors (of size 20 each) extracted to produce classes of chains. There is a matlab kmeans function.
- Classify the chains.
- The class chains form the input vocabulary to plsa, each character a document and each chain a word. Running plsa is now straightforward.
Summary
- I have accomplished the most important tasks I set out to do for the milestone, and I believe I am on track to produce character classification code in the next few weeks.
References
- Infty Project. http://www.inftyproject.org/
- Nakagawa, Nomura, Suzuki. Extraction of Logical Structures from Articles in Mathematics. http://www.springerlink.com/content/x4t3xc9l13pt6f5g/fulltext.pdf
- Belongie, Serge. Shape Matching and Object Recognition Using Shape Contexts. http://www.cs.berkeley.edu/~malik/papers/BMP-shape.pdf
- Hoffman, Thomas. Probabilistic Latent Semantic Analysis. http://citeseerx.ist.psu.edu/viewdoc/download?doi=10.1.1.84.1137&rep=rep1&type=pdf.